Digital Good and Evil
Responsible tech, and responsible AI governance in particular, defines the line between good and evil in a digital space. Unethical online behavior or online design is what harms people in some way.
Digital ethics dwells in the realm of the universal moral law, hardwired into each one of us – we call it conscience. We instinctively know right from wrong, even when we struggle to explain why.
- In Thomas Jefferson’s poetic prose of The Declaration of Independence:
We hold these truths to be self-evident, that all men are created equal, that they are endowed by their Creator with certain unalienable Rights, that among these are Life, Liberty and the Pursuit of Happiness. - In the Bible it is The Golden Rule:
Do unto others as you have others do unto you. - In the Hippocratic Oath of doctors:
First, Do No Harm. - In ancient Stoic philosophy it is living life in accordance with the virtues:
Wisdom, Temperance, Courage, and Justice.
Continuing this noble tradition, tech ethics is the discipline dealing with moral principles, what is good or bad, right or wrong, just or unjust, what humans ought to do. What is ethical and what is legal overlap, but they are not the same thing.
Legal vs Ethical
Legal regulations of online practices are just the set of rules written into law so far, often lagging decades behind newly emerging online harms. To be legal, tech companies do not need digital ethicists. They just need lawyers and compliance officers.
Let’s look at concrete examples of the complex interplay between legal and ethical.
Legal and Ethical
Tech giants claim to be ethical but their track record shows otherwise. The only tech companies that made it to Ethisphere’s list of most ethical companies in 2024 seem to be hardware makers: IBM, Dell, Hewlett Packard – whose business is not based on manipulating human psychology.
Legal and Unethical
A lot of what’s going on on the Internet today is perfectly legal and completely unethical. Giving 13-year old girls unfettered access to toxic social media like Instagram, that has been proven to cause anxiety, depression, and suicides, is perfectly legal, because the age of Internet adulthood is 13, according to an outdated 1998 Children’s Online Privacy Protection Rule (COPPA) law. Facebook whistleblower Frances Haugen revealed that Meta knows perfectly well what it does to young girls, but writes it off as collateral damage, and actively promotes damaging content in the name of user engagement and profit.
Tech executives who send their own children to device-free Waldorf schools but market addictive digital media to our kids are in clear violation of the Golden Rule: they do unto others what they protect their own children from.
Illegal and Unethical
These are the criminals. Drugs, human trafficking, child pornography, illegal gambling, financial scams – all are plentiful and easily scaled on the Internet. AI is currently busy supercharging this garbage – just scroll through your spam folder to see how.
Illegal and Ethical
One can envision such a category – when doing the right thing could be deemed illegal in the eyes of the law. For example, when a company blocks content it considers dangerous or false, it can face backlash for violating the freedom of speech. Twitter/X has done this before being acquired by Elon Musk when it suppressed some conservative voices. There is a thin line between user protection and totalitarian censorship. It’s a gray area. Every Trust and Safety department at the Big Tech will struggle with such moral dilemmas for years to come.
Ethical but potentially illegal practices might include polar opposite examples of content moderation (limiting damaging information), viewed as a violation of free speech, or whistleblowing (oversharing damaging information) by insiders who expose corporate dirty secrets to the public. Those who say society doesn’t punish these people should revisit the story of Edward Snowden.
Online Harms List
Any product could potentially cause harm in 2 ways:
- Intentional, built into a product (addictive drugs)
- Unintentional (negative side effects of life-saving drugs).
Most digital harms were initially in the unintentional category – tech companies did not mean for them to happen. But when companies like Facebook realized that these negative side effects come with high profits, they kept lethal design features in the code, fully aware of their harms to the user – like teen suicides. Like the tobacco companies of old who knew that smoking causes cancer, they continued to market their product to adolescents, knowing full well that Instagram causes mental health problems.
The creators of these products did not set out to increase teen suicide rates. Most of them were idealists seeking to make the world a better place. Technology was created to help humanity. But FDA approval was not required, and no clinical trials were conducted. Instead, we all found ourselves in the middle of a giant experiment by giving our default consent to use untested digital drugs. And the nasty side effects have manifested themselves in billions of users.
A non-exhaustive list of online harms has been put together by the Center for Humane Technology:
- Abnormal brain development in youth, mental health issues, social challenges, academic failure, cyberbullying, sleep deprivation, sexual predators, pornography exposure, self-harm
- Human downgrading, loss of attention and cognition
- Misinformation, conspiracy theories, fake news, propaganda, disrupted democratic process
- Physical and mental health: addiction, loneliness, stress, risky behavior
- Social relationships: less empathy, more confusion
- Systemic oppression, amplification of existing biases, injustice, violation of human rights
- Surveillance, coercion, operant conditioning and manipulation of humans
With AI amplification of harmful algorithms, the list is going to grow and the harms will become ever more catastrophic.
Data: Garbage In, Garbage Out
The algorithms are only as good as the data they train on. If the data is bad, we end up with biased and discriminatory AI. Examples abound: women are denied job interviews more than men, black people denied credit more than white people. The algorithm picks up variables it considers most predictive – which could be unethical, illegal, or both.
Sometimes AI also picks up accidental (spurious correlation) variables that have nothing to do with the prediction it’s trying to accomplish: in a well-known experiment University of Washington has “trained” an algorithm to distinguish wolves from huskies, only to discover that the algorithm was only paying attention to the snow in the background. The program was unusable if the animals were photographed in a different environment.
Debug the Design
If the algorithm is harming people, digital ethics considers it a social responsibility of the company to change the code and debug the compromising design. To tweak it until there are no negative side effects on the well-being of the user – even if it results in foregoing millions of dollars in profit.
And not just because it’s the right thing to do, but for the long-term survival of the company. The business’s very existence depends on avoiding catastrophic legal, financial, and reputational risks. At the time of this writing, Character.AI is facing a lawsuit because interactions with its chatbot led a teen to commit suicide. It would be exceedingly hard for them to maintain a successful business long-term while facing outrage from the customers, fines from the government, and multimillion dollar lawsuits from the lawyers.
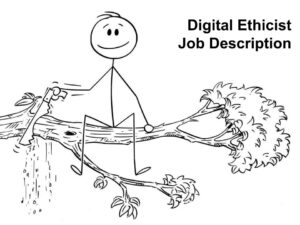
The Alignment Problem
The danger of AI lies in what is called the alignment problem – the behavior of AI becoming misaligned with the intentions of its human creators. When given a specific goal, algorithms figure out the most efficient way to achieve it, but often use methods that humans did not anticipate, resulting in dangerous unforeseen consequences. These are sometimes called externalities, or “revenge effects”. For example, social media algorithms figured out that outrage is the best way to maximize user engagement (the goal), started promoting fake news and conspiracy theories (the method), resulting in societal division and breakdown of democracy (revenge effect).
Philosophy of Self-Correction
To solve the alignment problem, strong self-correcting mechanisms need to be built to enable the algorithms to doubt their decisions and revise themselves. But how do we teach the machines what is the right thing to do? Unlike us, they are not conscious and do not have a human sense of right and wrong.
There are two philosophical arguments proposed as solutions, both imperfect. One is to use a set of universal moral principles, aligned with the Golden Rule. But it often breaks in reality because every group and society defines moral rules differently. The other approach is utilitarian: judge every algorithmic decision by calculating the amount of suffering or happiness it causes. This in turn is riddled with its own problems: the calculus of suffering is arbitrary and subjective, some stakeholders win while others lose. It’s difficult to determine if positives outweigh the negatives, leading us back to the search for universal truths.
Even if truth is often hard to find, it is a good idea for the algorithms to be guided by principles. By values. Or, as philosophers have been calling it for thousands of years – virtues. Virtues that have worked for humanity for a very long time. Honesty. Integrity. Accountability. Justice.
Algorithmic Justice
Yuval Noah Harari argues in his book Nexus: A Brief History of Information Networks, that for democracies to survive, the principles of information networks should be aligned with human well-being and include, at a minimum, these 4 components:
Benevolence
Access to our data comes with fiduciary responsibility to act in our best interest, like a family doctor or lawyer. Powerful tech tools in the hands of corporations or governments, acting in pursuit of their own aims, could lead to digital totalitarianism.
Decentralization
Information should not be concentrated in one place to avoid totalitarian control, but instead distributed among many nodes and networks that keep checks and balances on each other.
Mutuality
Watch the watchers. As data brokers collect information about the people, they are also accountable to the people in the system of mutual surveillance. The work of the algorithms is transparent and explainable.
Change and Rest
Throughout history oppression took the form of either denying humans the ability to change by forcing them into rigid categories, or denying humans the opportunity to rest by forcing them to change constantly in defiance of human nature. Harari writes: “Human life is a balancing act between endeavoring to improve ourselves and accepting who we are”. We need to prevent the algorithms from becoming too rigid or too demanding, and respect our biological rhythms instead.
AI Ethics Principles
2020 Harvard AI Ethics report identified 8 common AI ethics principles from existing corporate practices:
- Privacy
- Accountability
- Safety and security
- Transparency and explainability
- Fairness and non-discrimination
- Human control
- Professional responsibility (ethical and legal concerns in process and organization)
- Promotion of human values (are your actions in service of human flourishing)
Adherence to these principles is a good standard to evaluate against when building an AI system. At the moment, being ethical is voluntary. In the future, it is highly likely that these principles will become the law, so it’s a sound business practice to build a compliance network around them in advance.
Transparency
Transparency is about trust. We can trust the decisions of the machine only if we know how it made the decisions. How does the algorithm make predictions that affect human lives? Where did the training data come from? Is the decision fair? What are the motives of the agent using an AI system? Is there a conflict of interest that makes them violate human rights in some way?
Reid Blackman, the author of the book Ethical Machines, thinks that a company owes people an explanation when the decision made upon the recommendation of an AI harms them in some way. For instance, when deciding who gets a loan, an interview, or insurance, AI transparency would ensure the system is fair.
If a transparency check reveals some unavoidable negative side effects of the algorithm, these online harms should be disclosed. Disclosure of potential risks is the standard practice for physical products from furniture to drugs. Drug regulations require that every medicine must list potential side effects, and there is an agency that prevents truly harmful products from ever reaching the market: FDA. Cigarette packs come with a warning about cancer. Buyer beware messages for social media would include mandatory warnings about addiction, anxiety, depression, and suicide. Scroll responsibly.
AI transparency is people understanding how algorithms work. If AI is a black box, it is not defensible. But explaining how AI works is not an easy task.
Explainability
AI explainability is the key to tech ethics – why did the algorithm arrive at a certain decision. Complex neural networks are incomprehensible even to their creators, but if the decision of the algorithm cannot be explained in human terms, it cannot be justified. If explainability requires a sacrifice of predictive power and makes the tool less accurate, it’s a sacrifice that companies might need to make. The companies that use algorithmic black boxes expose themselves to catastrophic legal, reputational and financial risks. Unexplainable AI is a lawsuit and a compliance disaster waiting to happen.
It’s impossible to eliminate data bias entirely because what data does is divide people into categories using various variables. By definition, every data analysis is biased. What digital ethics can do is vet the data for discriminatory and problematic variables. Which is hard to do in practice, because the algorithms can assign a weight to billions of variables, most of which make no sense to the human observer, but might be deemed by the machine as predictive.
For example, for a hiring algorithm to be just, it can discriminate candidates on variables of merit (education, relevant experience), but not on variables of identity (race, gender), and should not take into account irrelevant correlations (like taste in music) that have predictive value but have either nothing to do with candidate’s performance, or contain hidden bias.
There is no way to figure out if a given variable is biased – machine logic is alien intelligence to us. A human could theoretically understand that a variable “zip code” could be a proxy for a race and therefore should not be used. But no human auditor could decipher a billion variables.
The workaround is to ask AI to explain its logic in human terms, or use a parallel AI audit system that could condense a billion numbers into a story that makes sense to a human. Without such a story, AI is not explainable and might be potentially harmful.
A good algorithm makes things fair: it will still sort people into categories but protect them from discrimination. If hidden biases are revealed inside the code, unlike prejudices and stereotypes in a human brain, these can be fixed, making an algorithm more impartial than a human decision maker. Nevertheless, a human should always be in the loop.
Human Oversight
To safeguard the technology, human oversight is essential. A standard practice in research is to use IRBs – Institutional Review Boards, whose job is to make sure no harm is caused to the human subjects.
The Belmont Report, generally accepted in all research as ethics standard, outlines three core ethical principles that guide research involving human subjects:
- Respect for persons (information, voluntariness, comprehension)
- Beneficence – do no harm, maximize benefits and minimize risks
- Justice – fair distribution of costs and benefits, potential impact on vulnerable groups.
The Belmont Report’s principles have been incorporated into “Common Rule” (45 CFR 46), which governs federally funded research involving human subjects. FDA regulations for studies are even stricter: if the investigator stands to profit, they need to disclose the conflict of interest.
Facebook, Twitter/X, Google, Tinder and others do not obtain any IRB permission to do their experiments on people. Millions of A/B tests are performed every day that potentially violate the principles of ethical research. The now infamous Facebook emotions study separated 700K users into 2 groups with either positive or negative words blocked in their feeds, and then followed to see if they have become more positive or negative. Facebook manipulated the emotions of users without asking their permission, violating several ethics principles: users were not informed, subjects were not respected, harm to emotional health was caused. Cambridge Analytica was unethical in its use of NLP (natural language processing) for personality recognition to identify the worst personality traits to brainwash and manipulate people.
To monitor the algorithms, human supervisors could be a Trust and Safety department, ethics committee, risk board, or anything else, and would have to be a cross-functional team, consisting of ethicists, psychologists, even philosophers, not just data scientists and lawyers.
The best ethical oversight committee should be what is called a “centaur”, like the best chess teams in the world – a team of humans and algorithms working together. People on the committee should come from a broad diversity of opinions across the entire political and social spectrum, have equal votes, and operate as a democracy. AI systems they use should have ethical parameters built in.
Current State of Affairs: Out of Control
BJ Fogg’s 2002 book Persuasive technology: Using computers to change what we think and do, had a chapter on ethics with urgent warnings about operant conditioning, surveillance, unintended outcomes, targeting vulnerable groups, and using emotions to persuade. The creators of tech products were his students at Stanford who used his insights to unleash technology on the world without ethical guardrails built in.
Online world is dishonest and manipulative. The Internet is full of mind control engineered to attract attention and drive traffic, messing with people’s fears. Conflicts of interest reign supreme. Opinions are ads in disguise. Surveillance and data mining fuels discrimination and algorithmic bias. Privacy is dead. Platforms are not accountable for the harms they cause.
A human brain has reached its limit in collision with AI. We can no longer control our destiny that is increasingly being determined by algorithms.
Cost/Benefit Analysis
Anywhere where there is a benefit to humans – like biometric trackers measuring our health and fitness – there is also an incentive to use this data for commercial gain. For now – with no restrictions. When we click “Agree to Terms and Conditions”, we sign our data away.
Personalized medicine is absolutely achievable, designed for us based on our genetic makeup and tracked history of our health, nutrition, activity, and biometric markers. We want that. There is a clear benefit. We are willing to share our data to make it happen.
What we don’t want is our insurance company to set our rate based on the same data set. The incentive is there to measure our health risk very accurately and price our insurance accordingly. Should the data of faulty genes or faulty lifestyle fall into their hands, they would be in position to discriminate based on biology and behavior.
Common business sense also dictates that insurance penalties for the unhealthy and unfit would exceed insurance discounts for the healthy and fit. Corporate greed is a permanent fixture.
Such power given to a commercial enterprise would be unethical and needs to be limited by law. Just like it’s illegal to discriminate based on race or gender, it should be illegal to discriminate on most of the data – biological or behavioral – collected from individuals.
Present day background checks routinely performed for job applicants have already expanded from criminal records and credit reports to include social media profiles. The standing recommendation is to clean up your social media profiles and adjust privacy settings to keep personal information from being accessed.
Data is from the past. Our past does not have to define our future. Because let’s face it – which one of us is without sin? We cannot win in a world where even a small transgression, committed decades ago, can be used against us. We are algorithmically set up for failure and rejection.
However, removing data risks violating freedom of speech. Digital ethics will need to determine the line where Internet regulation becomes censorship. That would be the end of democracy. Internet censorship is well established in countries like China and Russia.
Going Forward: Humane Technology
Big data algorithms need to be optimized for human well-being and positive social change – not just for profit and political gain. The goal of humane technologists is not neutrality. It is alignment: algorithms being beneficial to humanity.
AI designs that inform decisions in a complex environment should be guided by values such as overall well-being over a metric like user engagement. Values could be derived from user feedback about how using the product makes them feel, how it affects their relationships, health, and sense of self. Analysis of such feedback might rely on cross-disciplinary knowledge from psychology, social science, even philosophy, and could utilize natural language processing for efficiency. The intention is to optimize an AI system to be a positive force in the world.
It is probably naive to hope that in the near future the legal and the ethical in the digital space would converge, Compliance and Trust & Safety would be the same department, and the Internet would become a safe and beautiful place for all of us. But it’s an ideal to strive for.